Multi-Object Tracking with Hallucinated and Unlabeled Videos
Presented at CVPR 2021 LUV Workshop
-
Daniel McKee
UIUC -
Bing Shuai
AWS -
Andrew Berneshawi
AWS -
Manchen Wang
AWS -
Davide Modolo
AWS -
Svetlana Lazebnik
UIUC -
Joseph Tighe
AWS
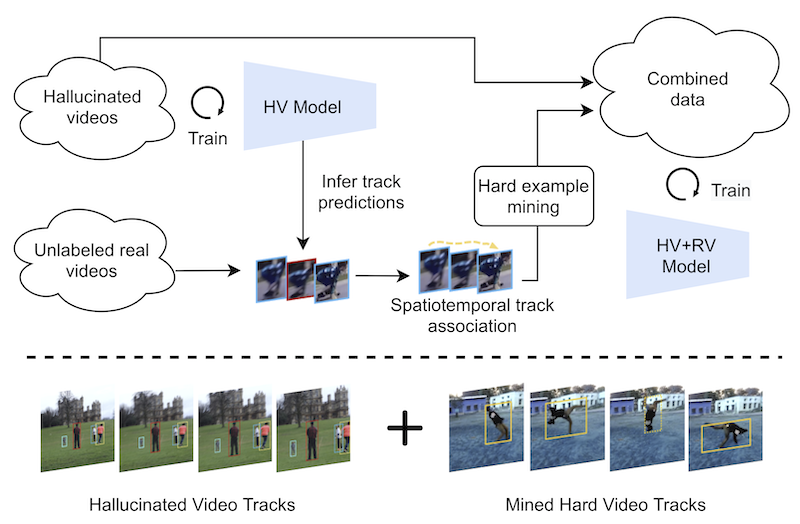
Abstract
In this paper, we explore learning end-to-end deep neural trackers without tracking annotations. This is important as large-scale training data is essential for training deep neural trackers while tracking annotations are expensive to acquire. In place of tracking annotations, we first hallucinate videos from images with bounding box annotations using zoom-in/out motion transformations to obtain free tracking labels. We add video simulation augmentations to create a diverse tracking dataset, albeit with simple motion. Next, to tackle harder tracking cases, we mine hard examples across an unlabeled pool of real videos with a tracker trained on our hallucinated video data. For hard example mining, we propose an optimization-based connecting process to first identify and then rectify hard examples from the pool of unlabeled videos. Finally, we train our tracker jointly on hallucinated data and mined hard video examples. Our weakly supervised tracker achieves state-of-the-art performance on the MOT17 and TAO-person datasets. On MOT17, we further demonstrate that the combination of our self-generated data and the existing manually-annotated data leads to additional improvements.
Video
Citation
Website template credit: Michaƫl Gharbi and Jon Barron